Top datasets used to train AI models and benchmark how the technology has progressed over time are riddled with labeling errors, a study shows.
Data is a vital resource in teaching machines how to complete specific tasks, whether that's identifying different species of plants or automatically generating captions. Most neural networks are spoon-fed lots and lots of annotated samples before they can learn common patterns in data.
But these labels aren’t always correct; training machines using error-prone datasets can decrease their performance or accuracy. In the aforementioned study, led by MIT, analysts combed through ten popular datasets that have been cited more than 100,000 times in academic papers and found that on average 3.4 per cent of the samples are wrongly labelled.
See
Turns out humans are leading AI systems astray because we can't agree on labeling#
technologyv #
AI #
OpenStandards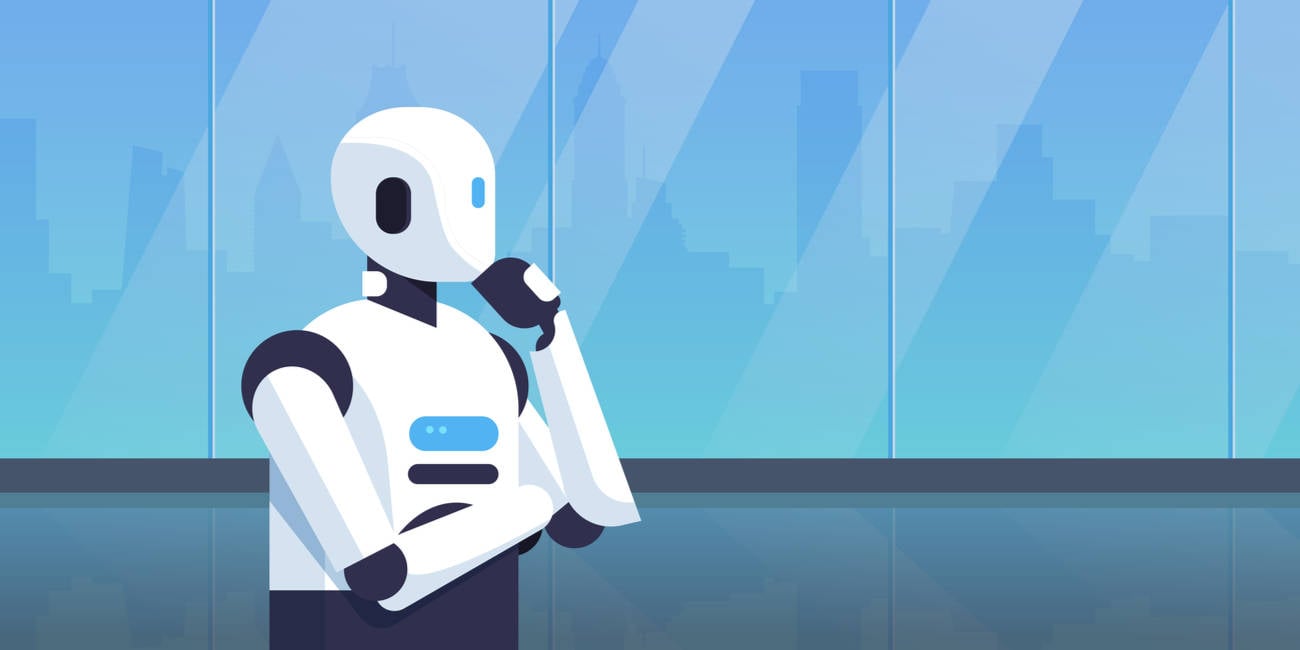
Is it a bird? Is it a plane? Asking for a friend's machine-learning code